We read every piece of feedback, and take your input very seriously.
To see all available qualifiers, see our documentation.
Have a question about this project? Sign up for a free GitHub account to open an issue and contact its maintainers and the community.
By clicking “Sign up for GitHub”, you agree to our terms of service and privacy statement. We’ll occasionally send you account related emails.
Already on GitHub? Sign in to your account
Is it possible to replace the existing Spark.NET with one that takes Spark scalar/java codes or Jars and compile that to .NET using IKVM?
@wwasabi
I know this is outside your scope, CC you as community here could start investigating ikvm
PySpark is a Python API for Apache Spark which is a data processing framework. The Spark core is implemented by Scala and Java, but it also provides different wrappers including Python (PySpark), R (SparkR), and SQL (Spark SQL). You can install Spark separately (which would include all of the wrappers), or install Python version only by using pip or conda1.
SparkR is an R package that provides a light-weight frontend to use Apache Spark from R. It is similar to PySpark but for R users1.
Spark.NET is a .NET library for Apache Spark which allows you to write Spark applications using .NET languages such as C# and F#2.
SparkR is an official Spark library while sparklyr is created by the RStudio community1. Due to the fact that currently Python is a favorite language for Data Scientists using Spark, Spark R libraries are evolving at a slower pace and in general catch-up with the functionality available in PySpark1.
sparklyr is an R package developed by RStudio folks and provides a complete dplyr backend to Spark, using the same dplyr syntax. That implies that switching between environments does not require changing of function names. In contrast to SparkR, here we operate on tables/tibbles, which are mapped to Spark DataFrames1.
https://spark.rstudio.com/
The text was updated successfully, but these errors were encountered:
No branches or pull requests
Motivation
Is it possible to replace the existing Spark.NET with one that takes Spark scalar/java codes or Jars and compile that to .NET using IKVM?
@wwasabi
ChatGPT reply
PySpark is a Python API for Apache Spark which is a data processing framework. The Spark core is implemented by Scala and Java, but it also provides different wrappers including Python (PySpark), R (SparkR), and SQL (Spark SQL). You can install Spark separately (which would include all of the wrappers), or install Python version only by using pip or conda1.
SparkR is an R package that provides a light-weight frontend to use Apache Spark from R. It is similar to PySpark but for R users1.
Spark.NET is a .NET library for Apache Spark which allows you to write Spark applications using .NET languages such as C# and F#2.
SparkR versus Sparklyr
SparkR is an official Spark library while sparklyr is created by the RStudio community1. Due to the fact that currently Python is a favorite language for Data Scientists using Spark, Spark R libraries are evolving at a slower pace and in general catch-up with the functionality available in PySpark1.
sparklyr is an R package developed by RStudio folks and provides a complete dplyr backend to Spark, using the same dplyr syntax. That implies that switching between environments does not require changing of function names. In contrast to SparkR, here we operate on tables/tibbles, which are mapped to Spark DataFrames1.
https://spark.rstudio.com/
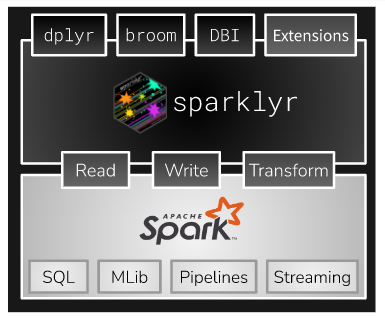
The text was updated successfully, but these errors were encountered: